The Potential of Independent Data Layers in IoT for Commercial Real Estate
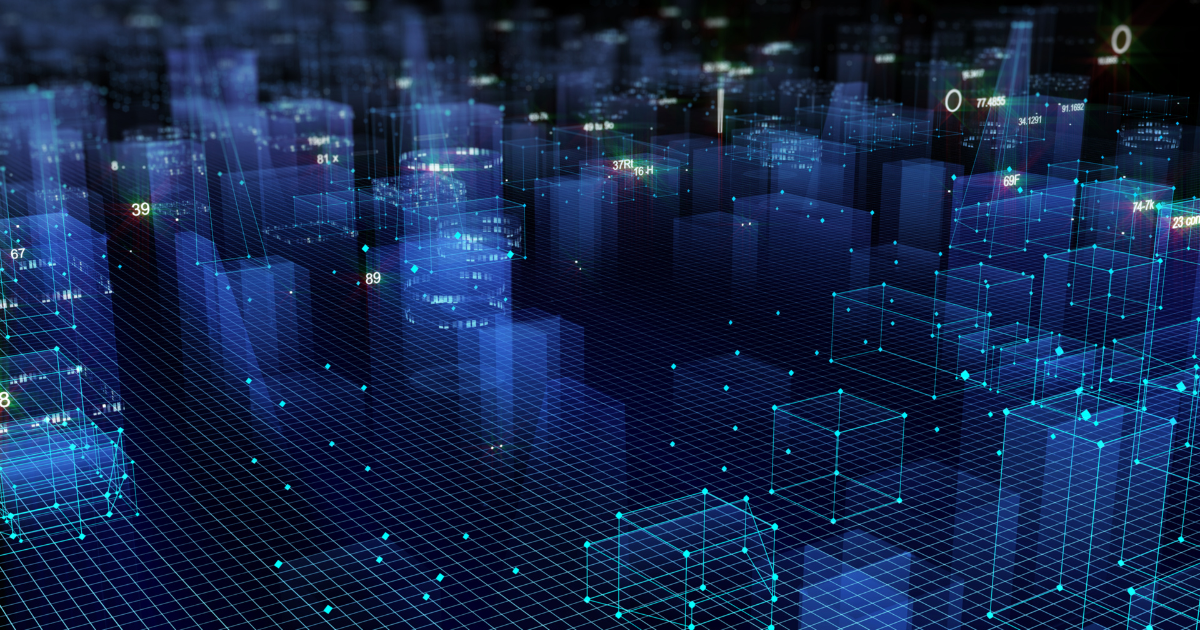
In the rapidly evolving landscape of commercial real estate, the effective management and utilization of data have become pivotal to success. To meet the growing demands of the industry, innovative solutions are required. One such solution is the concept of an independent data layer, as exemplified by Mapped, which acts as a bridge between the complex web of building systems and the needs of various entities within the commercial real estate sector.
Independent Data Layer
An independent data layer occupies a unique position in the realm of IoT (Internet of Things) for commercial real estate. It serves as an intermediary between the numerous data-producing systems within a building, such as HVAC, Lighting or Occupancy Sensors, and the individuals / applications that require access to this valuable information. In essence, the IDL acts as a translator, extracting, normalizing, and organizing data from diverse building systems, making it easily accessible for various applications and analytics tools.
It's essential to underscore the "independent" aspect of the "independent data layer," as many systems rely on proprietary data layers that can lead to vendor lock-in. By embracing a genuinely independent data layer, various platforms and systems can be employed without the need to overhaul the discovery and ingress methods at the building level.
Discovery and Ingress
At the core of an IDL is its ability to discover and ingest data from on-premises building systems. This process is facilitated by a gateway, which can typically be deployed in multiple ways to suit the specific needs of a building. With Mapped, it can be a physical hardware gateway or a software agent deployed within the building's network environment.
This gateway should be able to operate in both passive and active modes, in order to collect data efficiently. In passive mode, it listens to data traffic on the building network, enabling the discovery of various systems and their components. In active mode, the gateway actively polls devices to retrieve new data values. This flexibility ensures the IDL can adapt to different building network infrastructures, even those with older equipment.
Security is a paramount concern for any IDL, as collecting data from building systems can be sensitive. Mapped addresses this by implementing robust security measures, including secure boot, firmware signing, hardware enclaves, and encryption through mutual Transport Layer Security (TLS).
In addition to collecting data from building networks, an IDL should be able to integrate with various cloud APIs to harvest data directly from vendor systems. This approach, either in combination with a local gateway or independently, allows for comprehensive data acquisition, ensuring that all relevant information is accessible through the platform.
Normalization and the Power of the Graph
While just retrieving the data is of high value, saving hours of manual effort, an IDL's true strength lies in its ability to normalize and organize data into a structured format. Mapped achieves this using the Brick schema. This schema-based approach allows Mapped to create a "living" graph that represents unifies all building assets into one standard, normalized graph. This graph provides a unified view of the building and its systems, making it easier to derive insights and relationships among various components.
Machine learning (ML) could also play a critical role in the data normalization process. ML algorithms are employed by Mapped to classify and interpret data, especially point names that may be cryptic or inconsistent across different systems. This intelligent classification aids in accurate data mapping and normalization, increasing the value of the IDL.
Mapped's ML models further enhance data interpretation by discovering relationships between entities within a building. These relationships include compositional, functional, and geographical connections, allowing for a comprehensive understanding of the building's dynamics.
Accessing the Data
After extracting and normalizing building data, you’ll need a way to access it. The IDL will typically need to provide both pull and push methods. For example, developers and users can interact with the Mapped platform through its GraphQL API. GraphQL allows users to request (pull) precisely the data they need, making data retrieval efficient and tailored to specific requirements. There are also integrations with external platforms, like Microsoft Dynamics 365 for work order management, or with external data ingestion services like Azure Event Hub.
Conclusion
In the fast-paced world of commercial real estate, data is a precious commodity. An independent data layer revolutionizes the way data is collected, organized, and accessed within buildings. By providing a unified, structured, and secure platform, the IDL empowers various stakeholders to make data-driven decisions, derive valuable insights, and drive innovation in the industry.
This Week’s Sponsor
Mapped is a Delaware corp. headquartered in El Segundo, CA. Founded in 2019 by Shaun Cooley (Ex Vice President and CTO of Cisco IoT). The company is venture backed by leading industry VCs and corporates like Greycroft, MetaProp, Honeywell, Allegion, among others. Our employees are based across the US, Ukraine, Germany, Brazil, Portugal, and Poland.
Read Next
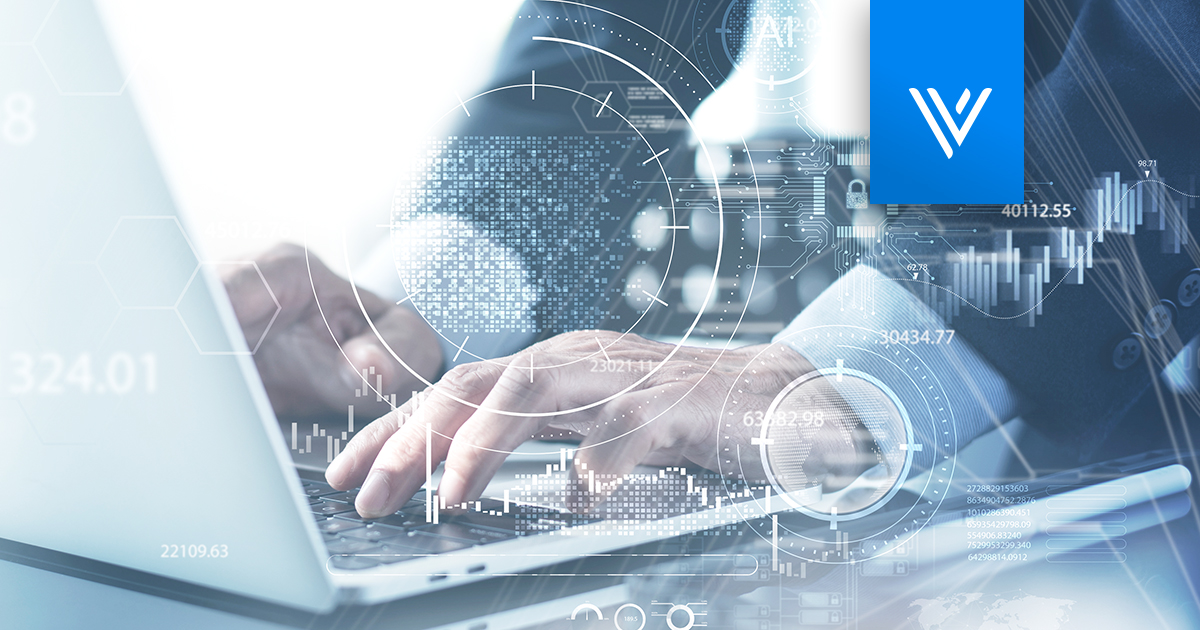
Putting the Power of AI to Work for CRE The business case for AI in commercial real estate is coming into clear focus. When combined with deep operational data,
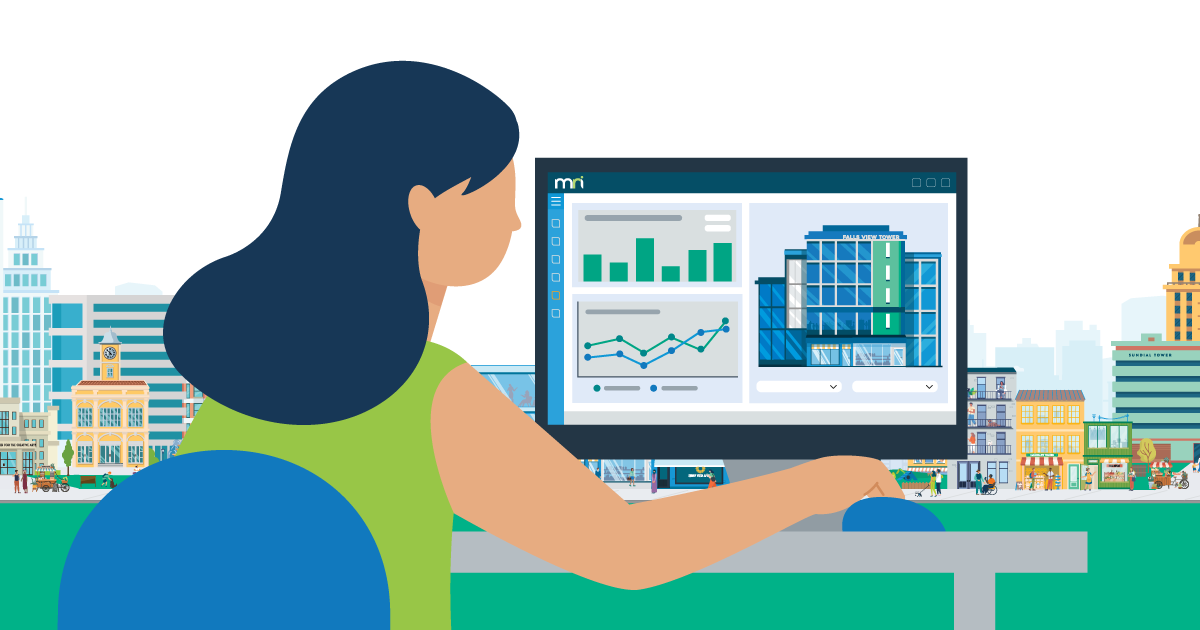
Economic Occupancy: Measure the Effectiveness of Your Real Estate Investments Economic occupancy is a crucial metric for measuring how effectively you manage and invest in real estate
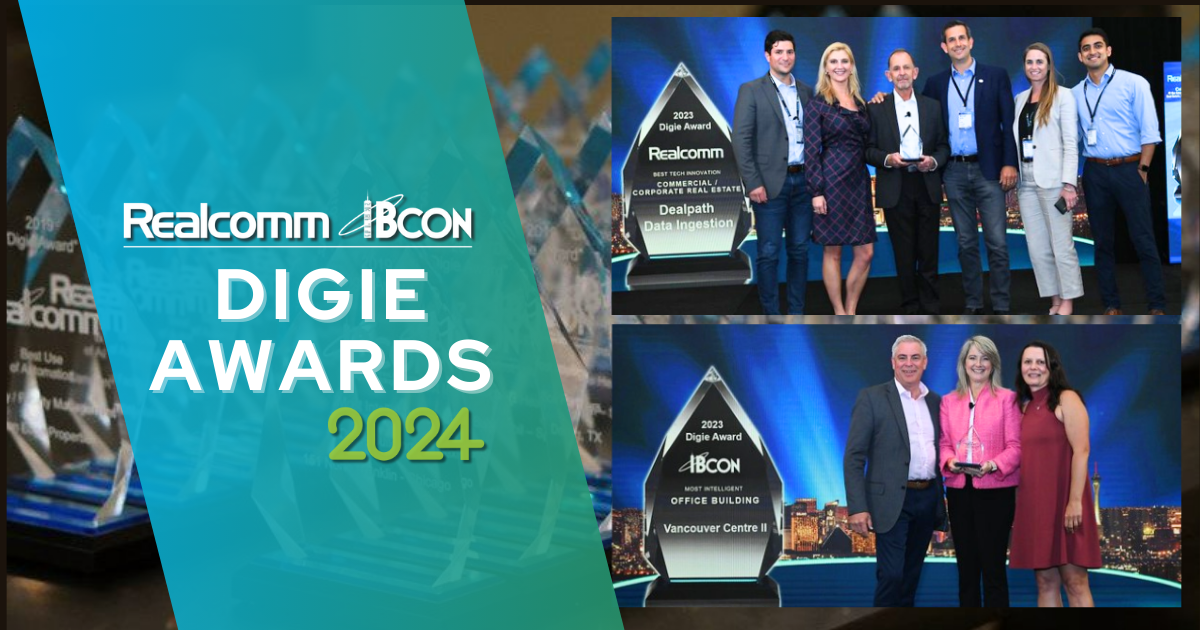
26th Annual Realcomm | IBcon Digie Award Finalists Announced! Realcomm has been presenting the Digie (short for Commercial Real Estate Digital Innovation) Awards since 1999...
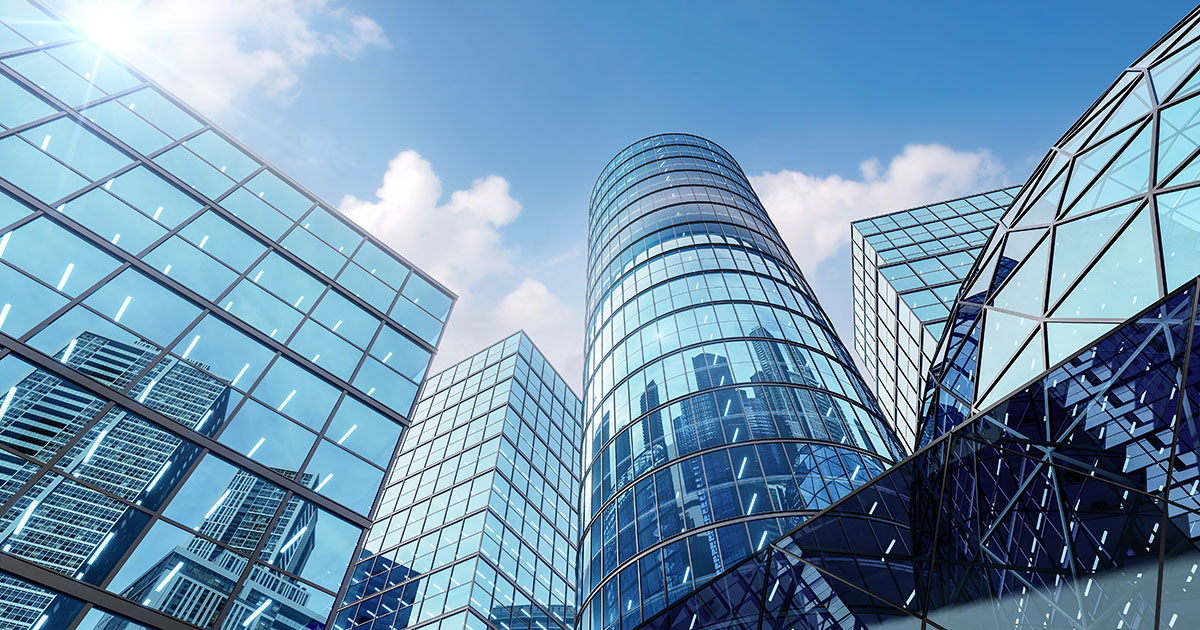
The Unrealized Value of Smart Building Systems Building owners have invested in smart buildings based on the promise that bringing diverse building systems together for data sharing and management will reduce operational costs and make assets more attractive to tenants.